Last update: 2019, Jan. 7
What is Marine Data Science (MDS)?
MDS is all about uncovering findings from marine data such as oceanographical data collected using both in situ methods and remote sensing. Typical parameters measured on-board relate to near-surface meteorological conditions (air temperature, wind speed, wind direction, cloudiness, etc.), sea surface conditions (e.g. sea surface temperature (SST), salinity, wave height, and wave direction) as well as subsurface water characteristics (vertical profiles of temperature, salinity, dissolved nutrients, ocean currents, or ocean bottom depth). Marine biological data comprises of spatial and temporal species occurrence and abundance data (e.g., trawl data of fish, net sampling of plankton, benthic grab samples or visual counts of mammals or sea birds), taxonomic information, trait data, sequence and high-throughput screening (HTS) data, as well as digital image data from optical sensor systems or hydroacoustic data from echo sounders.
When given a challenging question, data scientists become detectives and investigate leads and try to understand patterns within the data. It typically starts with data exploration followed by quantitative techniques drawn from mathematics, statistics, information science, and computer science in order to get a level deeper, e.g., inferential models, segmentation analysis, time series forecasting or synthetic control experiments. The overall intent is to scientifically piece together a forensic view of what the data is really saying about marine system dynamics. The data to be used, however, does not need to represent exclusively marine biotic and abiotic data. In fact, any type of data describing a climatological, terrestrial or socio-economic component that could affect the marine system could be analysed. Amongst these, regional and large-scale climatological indices, fisheries, agricultural, and demographic data are commonly used to study the extent of external forcing on the system and potential feedback mechanisms.
With the vast amount of data that are nowadays produced (~90 percent of the data in the world today has been created in the past two years1), twenty-first century marine science becomes increasingly analytical and computational across all disciplines. Since 2012, data science has become a popular buzzword when the Harvard Business Review called it “The Sexiest Job of the 21st Century”2 and is often used synonymously with statistics although it comprises of more disciplines.
Elements of data science
Data science is highly interdisciplinary and a blend of skills in three major areas:
Hence, new generations of marine scientists need to be well trained in
- analytical and interdisciplinary thinking
- computer programming → at least one language
- visualizations
- data mining, statistical modelling, machine learning, and predictive analytics
Popular data science methods
The online community platform of data scientists and machine learners kaggle conducted in 2017 an industry-wide survey amongst 16,000 data scientist from all over the world. The respondents were asked about their educational background, working environment and programming languages and methods used at work or in the near future. You can find a summery of the survey here https://www.kaggle.com/surveys/2017 and the complete survey results here https://www.kaggle.com/amberthomas/kaggle-2017-survey-results. The data can also be download at https://www.kaggle.com/kaggle/kaggle-survey-2017.
Let’s see which methods respondents from all fields or academia currently use most at work:
p_all <- methods_now %>% ggplot(aes(x = selections, y = percent, fill = selections)) +
geom_col() + scale_fill_viridis_d(direction = -1) + guides(fill = "none") +
labs(x = "Methods used", y = "Percent") + coord_flip() + theme_classic()
p_acad <- methods_now_acad %>% ggplot(aes(x = selections, y = percent, fill = selections)) +
geom_col() + scale_fill_viridis_d(direction = -1) + guides(fill = "none") +
labs(x = "Methods used", y = "Percent") + coord_flip() + theme_classic()
gridExtra::grid.arrange(p_all, p_acad, ncol = 2)
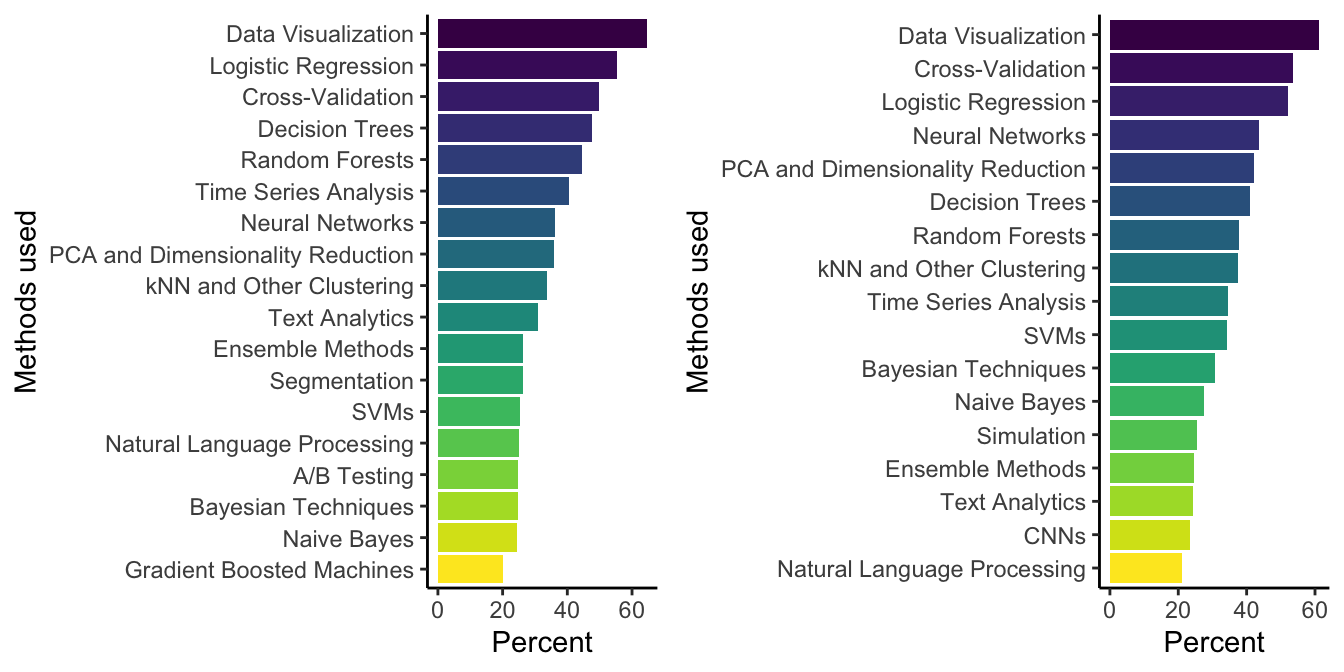
Figure 1: Barplot of most common methods amongst data scientists in all working environments (left) and in academia only (right).
Beside data visualization, logistic regression and cross-validation are the most commonly used data science methods, also in academia.
Which machine learning or data science method are data scientists most excited about learning in the next year?
Methods | All (%) | Academia (%) |
---|---|---|
Deep learning | 40.3 | 41.1 |
Neural Nets | 12.8 | 10.3 |
Time Series Analysis | 6.3 | 6.7 |
Bayesian Methods | 4.7 | 5.8 |
Text Mining | 4.6 | 5.1 |
Genetic & Evolutionary Algorithms | 3.9 | 3.3 |
Social Network Analysis | 3.4 | 3.5 |
Anomaly Detection | 2.8 | 2.5 |
Ensemble Methods (e.g. boosting, bagging) | 2.5 | 2.5 |
Other | 2.4 | 2.9 |
Monte Carlo Methods | 2.1 | 2.5 |
Cluster Analysis | 2.0 | 2.4 |
Regression | 2.0 | 1.2 |
I don’t plan on learning a new ML/DS method | 1.8 | 2.3 |
Decision Trees | 1.4 | 1.1 |
Random Forests | 1.4 | 1.1 |
Support Vector Machines (SVM) | 1.4 | 1.4 |
Survival Analysis | 1.0 | 1.1 |
Proprietary Algorithms | 0.9 | 0.9 |
Factor Analysis | 0.7 | 0.6 |
Link Analysis | 0.5 | 0.4 |
Association Rules | 0.4 | 0.7 |
Rule Induction | 0.4 | 0.5 |
Uplift Modeling | 0.3 | 0.0 |
MARS | 0.1 | 0.1 |
Independent of the working environment, deep learning and neural networks are by far the methods data scientists are most excited to learn.
Report at the IBM Consumer Products Industry↩
Davenport, T.H. & Patil, D.J. (Oct 2012), Data Scientist: The Sexiest Job of the 21st Century, Harvard Business Review↩